Our value proposition
Data Product Strategy
A strategy and vision for driving digital transformation through data and AI revises the current strategy and vision of the company and readjusts it to the new realities in the industry. It addresses the four distinct perspectives (strategy, product, capabilities and transformation).
DEFINE STRATEGY & METRICS
How is your industry affected through data and AI? Does your current business model and corporate strategy need to be adapted? What should be key metrics?
ASSESS TECH & ORG
Which capabilities and technologies are needed to deliver the vision and strategy? Is the current architecture and organization able to deliver it?
DESIGN PRODUCT PORTFOLIO
In which business areas and for which purposes should data products be designed and developed to best support the vision and strategy?
BUILD DATA & AI ROADMAP
What are the business changes needed to implement the strategy and vision? How can the transformation of the company be managed? What are the steps on the way?
Best practice
Data and ML Ops
The machine learning model needs to be supplied regularly or even constantly with fresh data, which is like oxygen for a data product. The data pipelines are the blood veins that transport the oxygen that need to be monitored and quality controlled 24/7 by building software scripts for data quality profiling with business rules and anomaly detection. The monitoring should provide warnings if data is not loaded correctly, data formats do not match the expectations, the content of data appears to be unusual. Machine learning models need to be re-trained with new data regularly to ensure that they capture systematic changes and incorporates new features or business logic adaptations (such as the rise of the electric vehicles in the automotive sector). Monitoring also needs to be applied to the entire software code and infrastructure to ensure a smooth operation and fast mitigation of problems.
Best practice
Scaling data products
Data products can often be scaled and rolled out to other business scopes to increase business value, which is done in the data product scaling phase. This would include the adaptation of data products to other parts of the business and expanding the functionality of data products to solve more business problems in the same business domain. Scaling a data product to a new scope usually requires an adaptation of the data product to the new scope. For example, data models and the business logic might be different in another production plant or market, and, hence, the model features, machine learning models and data preprocessing pipelines have to be changed accordingly to make the data product product usable in the new scope. In many cases, there are substantial synergies when scaling data products and, therefore, value can be generated faster, eg when features and target variables for machine learning models are the same.
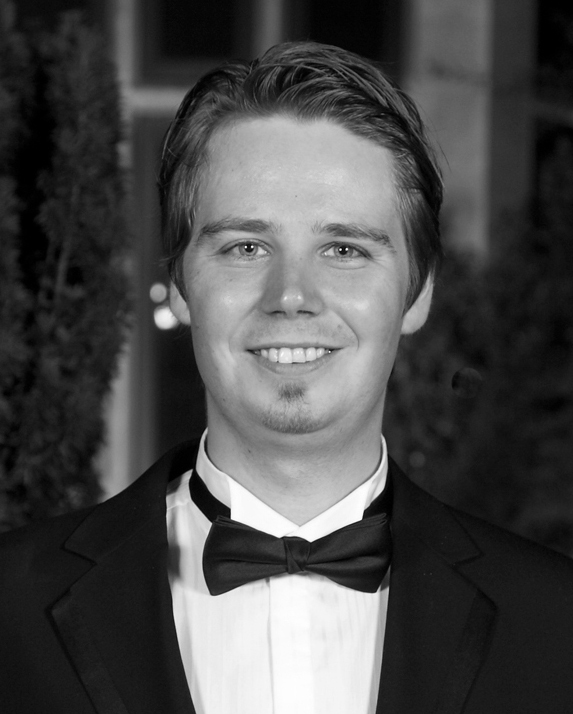
Leadership
Dr. Alexander Borek is an experienced data and AI leader, author and keynoter and supported many tech and established companies on their data journeys, in particular, with regards to strategy, roadmap, product portfolio and architecture. He brings more than a decade of experience in successfully establishing 15+ global data platforms and organizations and developing and scaling >50 impactful machine learning and analytics products.
In his previous role, he served as the Global Head of Data, Analytics & AI at Volkswagen Financial Services, which provides the financial products for the Automotive Brands of the Volkswagen Group. He ran an internationally operating unit consisting of ML experts, data scientists, cloud architects, front and backend developers, data engineers, product managers and UX designers to develop and operate high-end data products.
Our books
Covering all data topics
CASE STUDY
Smart logistics
Supporting a leading logistics tech company in their data transformation journey
DATA PRODUCTS
Design and delivery of data products for pricing and network optimization
AGILE ORGANIZATION
Setting up autonomous mission driven cross functional product teams with OKR steering
DATA PLATFORM
Implementation of cloud based data platform and data governance
ANALYTICS ROLLOUT
Rolling out central analytics tool and dashboards to all functions and across markets
Got interested?
Leave us a message with your question